DYNAMIC BRAIN PROCESSES OF READING COMPREHENSION
Reading comprehension (RC) is a complex neurobiological process. To comprehend a text, a reader’s brain needs to rapidly and flexibly coordinate communication across widespread brain networks. This communication allows readers to map a symbol to a sound, link the sound-symbol to its meaning, unite meaning across words and sentences, and ultimately build an internal representation of a text (i.e. a “situation model”). The ultimate goal of RC is to then encode relevant aspects of the situation model into long-term memory (i.e. transform “comprehension” to “learning”). Learning from texts is one of the most complex forms of cognition, and presents unique methodological challenges. Brain research is one key avenue in which to characterize RC: research has allowed for the identification of “hidden” RC processes that are not always captured by behavioral tests, and which are strongly predictive of RC outcomes. In some cases, these brain patterns are predictive of reading outcomes even in the absence of behavioral differences. One key data challenge facing progress in brain-based RC characterization and enhancement is the need for high-definition neuroimaging to track dynamic RC processes across different types of texts. To capture the full complexity of RC, our work examines rapid brain network changes during RC and how they relate to long-term learning.
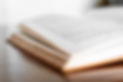
READING COMPREHENSION IN THE BRAIN
What are the real-time brain networks of RC? While the brain networks responsible for RC operate on the millisecond (or less) level, brain imaging has previously been unable to keep up: MRI can tell us where processes occur and EEG can tell us when processes occur, however no single modality can tell us both where and when processes occur in a data-driven manner. Addressing this challenge requires the unique use of different types of high-dimensional neuroimaging data and data reduction processes to identify meaningful real-time brain signals. My dissertation work directly addressed this challenge. By using a fused independent component analysis of MRI and EEG, we are able to track adult RC brain networks at a millisecond level. We've found that in typical adults, sentence comprehension involves rapid trade-offs between widespread brain networks related to meaning access, meaning integration, memory schema, semantic/syntactic re-appraisal, and conceptual coherence. This all takes place within one second of the sentence-final word. This work is the first to apply a fused MRI-EEG approach to language comprehension, and resulted in the most spatially and temporally refined tracking of language processes to date.
Our current projects use the fused MRI-EEG analysis to track real-time brain signals of RC over the course of a text. This research specifically focuses on how these signals change, and how those changes relate to reader- and text-based characteristics in adult readers.
LEARNING FROM TEXTS
What are the real-time brain networks of long-term learning from texts? Another limitation in the neurobiological study of reading comprehension (RC) is the failure to differentiate between RC and learning. While readers may be able to build a situation model while reading (“comprehension”), this may not result in long-term memory retention (“learning”). Previous work has demonstrated that texts with increased learning demand have different dynamic brain signatures than texts with lower learning demand. However, these projects do not directly examine brain signatures of learning (i.e. which brain areas successfully encode information into long-term memory), and do not use high-definition neuroimaging to track learning processes.
Our current research identifies the rapid brain dynamics of adults as they learn new information from medical texts. Rather than a focus on a reader’s general RC ability (see below), this project focuses on an individual’s real-time brain signatures of learning. By collecting a reader’s short- and long-term recollection of factual information, we are able to go back to the individual’s high-definition MRI-EEG signature at the time he/she was reading the learned information. We are then able to compare that to time points in which the individual failed to retain information. This research direction has the potential to identify individual-specific brain markers of learning for better prediction of learning outcomes, matching an individual to appropriate behavioral interventions, and generating targets for brain-based intervention.
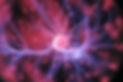